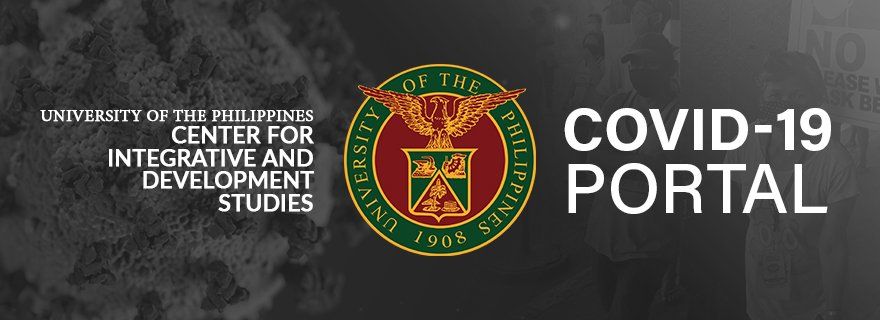
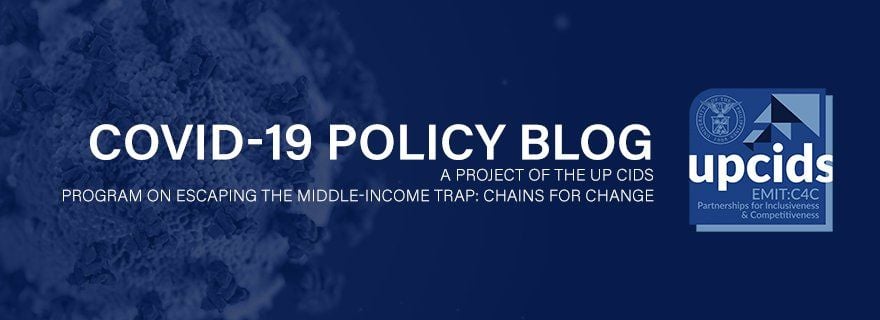
Post
Big data and AI as tools to fight the COVID-19 pandemic
Annette Balaoing-Pelkmans, Jane Lynn Capacio, and Tara Alessandra Abrina
How can big data and augmented intelligence help in the fight against the COVID-19 pandemic?

Key Points
- Big data and augmented intelligence (AI) are indispensable tools in the fight to overcome the COVID-19 pandemic.
- Each stage in a pandemic calls for shifts in the priority of a crisis response data and information system.
- There is an immediate need to streamline the flow of data for the purpose of addressing the most critical questions of the day. This would need establishing the knowledge value chain, from the collection of raw data, to the processing, and, finally, to data analysis to inform policy. Just as in any other value chains, this would entail building linkages, relationships, and good governance.
- The social and economic costs of the pandemic are mounting. Big data and AI are indispensable in getting our interventions right. Precisely in times of crisis, the deeply systemic nature of the problem requires systemic and evidence-based approaches.
The East Asian example: Big Data and AI for pre-pandemic preventive measures
The success of Taiwan, South Korea, Singapore, Hong Kong, and China in addressing the COVID-19 pandemic is being attributed not only to massive proactive testing, but also to the way these countries are able to use their arsenal of big data and augmented intelligence (AI) capabilities to trace, monitor, and contain the spread of the COVID-19 within a data management and analytics system.
Taiwan, for instance, exploited its national health insurance database and integrated it with its immigration and customs database to track the actual and potential path of the spread of COVID-19. It used real-time alerts during clinical visits based on travel history and clinical symptoms to facilitate case identification. QR codes were also used to ease tracking of travel history, with links to personal profile and individual health status and history. Through big data analytics, Taiwan was able to cluster their population into low- or high-risk segments based on their travel history and exposure to known cases. Those flagged as being high-risk were then quarantined and tracked through mobile phone data. A toll-free number was provided to enable reporting of health status and movement history, and this constant flow of information was then fed to the country’s big data platform.
China likewise unleashed their own battery of big data and AI instruments such as thermal scanners, passenger trackers based on real name systems, facial recognition, AI-integrated solutions,4 information from 300 million security cameras, GPS tracking of travel history, and surveillance drones. Mobile phone apps (i.e., close contact detector), and QR codes were also used to facilitate encoding personal information.
The cases of Taiwan and China amply show the indispensable role of big data and machine learning for combatting the COVID-19 pandemic. Through these means, public authorities are able to: provide real-time forecasts to inform policymakers as well as the general public; allow for the targeting of hot transmission areas; monitor the health status of the most vulnerable segment of the population; provide critical information to frontline health workers; and guide the procurement and distribution of critical medical supplies.
The European example: Changing priorities of data management and use at varying stages of the pandemic
On the other hand, the experience of Italy, Spain, and Netherlands, where the pandemic crisis is now most severe, shows that the utility of data and information changes at varying stages of contagion. Contact tracing for the purpose of proactive and selective containment of disease is most important at the early, and preventive phase of the disease, but at a critical stage of contagion, lockdown measures are instead more effective. Even proactive testing is relatively less important around the peak of contagion, not only because the whole population is practically quarantined, but also because, even for rich countries such as the Netherlands, the acute scarcity of testing materials and health care personnel simply no longer allow it.
The Dutch, Spanish, and Italian cases demonstrate that at a critical contagion rate, the pressure and demand on health system resources hardly leaves room for a more preventive approach. An active alert system, such as that employed by Taiwan, was not even put in place in the Netherlands. The latest measure is for a whole household to go on quarantine once any member experiences a known COVID-19 symptom. In areas with an uncontrollable rate of contagion, such as Italy, the government is left with no choice but to employ draconian lockdown measures that cover the entire population. This effectively assumes that the whole household, city, or even the whole country is a red zone.
It is obvious that there is no one-size-fits-all policy prescription for addressing a pandemic; context, as well as the various stages of the disease would correspond to different sets of priorities and policy actions.
Use and importance of big data and AI at various critical COVID-19 phases
The World Health Organization (WHO) developed a six-phased approach to pandemics in order to facilitate the design of national preparedness and response plans.5 Phases 4 to 6 are said to be most critical where response and mitigation efforts are needed. Table 1 (below) enumerates the main uses of a data management and analytics system at critical COVID-19 phases; that is, from Phase 4 to 6, as well as in the post-peak stage.6
Pre-pandemic Stage. In the early-pandemic phase (WHO Phase 4), big data and AI instruments are most needed for the development of an effective real-time alert system directed towards both policymakers and the general population. Given unlimited resources as well as speedy testing procedures, the first-best approach is to give the population access to testing facilities, even at various points in time when individuals sense the risk of virus exposure. However, even the richest countries do not have these unlimited possibilities; thus, a contact tracing system is the next best alternative to universal and multiple testing access.
A process of classifying various degrees of risks is obviously indispensable in such an alert system. Assigning the right level of risks to individuals enables them to apply the appropriate degree of precaution; contact tracing is key in doing this. In Taiwan, for instance, a low-risk status implies no travel to high-risk transmission areas (so-called Alert 3 areas) and therefore minimum movement restrictions, while those with history of travel are flagged as being high-risk and were then subjected to strict quarantine and monitoring procedures (e.g., regular personal checks and tracking of mobile phone GPS).
The Italian, and now the US experiences have demonstrated the vulnerabilities of the health sector in times of a pandemic. The surge of demand for medical supplies and equipment is exceptional due to the sheer rate of spread of the disease. One clear lesson that many European countries have drawn from the gravity of the Italian crisis is therefore the need to scale up the speed and volume of sourcing and production. Efficient monitoring of existing capacities and identification of new suppliers need a good data and information system in place—even before the critical stages.
Pandemic Stage. The priority need that must be addressed by big data changes at the pandemic stage, or the WHO Phases 5 and 6. Especially when ICT resources are scarce and coordination capacities are strained, it is important to focus on what is needed the most. Given the absence of vaccines and molecularly targeted medicines, it is critical to understand what types of treatment would seem to work, especially for the first countries inflicted by the disease.
The deep uncertainties surrounding the pandemic also makes forecasting crucial. The question now dominating everyone’s minds is when the peak of the transmission would be reached. Addressing this not only helps to allay general anxiety, but also informs planning and policymaking across all sectors of society.
A lockdown policy in the pandemic stage makes contact scanning less important for case identification and implementation of containment measures. But in porous cases, partial or selective lock-down, the absence of mass testing will continue to make contact scanning critical in identifying which location and individuals that authorities must strictly monitor for quarantine compliance.
Post-peak Stage. The data and information aspect of crisis management will remain important at the post-peak stage of the pandemic given the possibility of a second wave of virus outbreak. It typically takes a year before vaccines are developed and clinically tested as safe for mass adoption. Prolonged lockdown has enormous economic costs which will inevitably lead to public unrest and clamour for its termination. The transition towards normality will still be replete with risks and should be managed by careful surveillance.
The ideal scenario is a rapid containment of disease without resorting to an economically and socially costly lockdown.7 It is therefore precisely at an early pandemic stage that big data and AI instruments are most critical. Taiwan’s success is said to be the result of the 17 years’ worth of preparation after the SARS 2003 outbreak claimed 181 lives and infected 668 others. Undoubtedly, the COVID-19 pandemic will stimulate countries to adapt structural measures to strengthen their resilience against future viral outbreaks. The task of data and information managers would therefore be to efficiently harvest the necessary data for evidence-based medicine and policymaking.
Emerging priorities
There is an immediate need to streamline the flow of data and information for the purpose of addressing the most critical questions of the day. This would need establishing the knowledge value chain, from the collection of raw data, to the processing and finally to data analysis to inform policy. Just as in any other value chains, this would entail building linkages, relationships, and good governance. It would have to coordinate the whole chain of data collectors, data architects and engineers, scientists and researchers, policymakers, and even the public as the main source of data. The issue of governance is important because with the effort involving multiple parties, with different expertise, and often different focus, interests, protocols, there is a need for someone/agency to orchestrate it. A powerful mandate must be given for this person/agency to bring so many different sectors/experts together. The more systemic a problem is, the more important it is to bring the system (i.e., systemic players) in the room.
Health costs. There are urgent questions pertaining to the health aspects of the COVID-19 crisis that should be addressed by data management and analytics. How could we further improve the contact tracing of confirmed or potential COVID-19 patients? There are also questions on how to manage the health care value chain system to track the optimization of the distribution of critical resources (e.g., PPE, support for health front-liners). What is the current capacity of our health system and how can we address the weakest links, such as the ICU capacities and facilities?
Socio-economic costs. However, as the pandemic progresses, the social and economic costs are now becoming acute as well. The data and knowledge chain is indispensable in getting our interventions right in the whole area of social support for the most vulnerable segment of the population. Various public and private relief agencies are now targeting millions of people for immediate food support. But given the uncertainties as to the length of the pandemic, the issue of sustaining relief operations must be faced squarely. Given scarce resources, attention must be given, for instance, to the poor who have been infected and must therefore be quarantined. Especially, if these are bread-winners, it will be difficult to enforce their quarantine if their household’s basic needs for food are not addressed. A more targeted relief and social support system needs to be directed by good information as well.
MSMEs. The impact on micro, small, and medium enterprises (MSMEs) is staggering. There is now an overwhelming clamour for assistance, and yet, even here, policymakers must resist overly hasty measures and aim for an intelligent financial assistance system instead. Precisely in times of crisis, the deeply systemic nature of the problem requires a systemic and evidence-based approach.
Supply chains. Another critical area is to ensure the flow of critical goods and services. Keeping the Luzon-Visayas-Mindanao corridor for essential agri-fisheries products for instance, is an absolute priority. Through Big Data and AI we need to identify the “safe” routes, and also to certify that the sources of these products are likewise safe in order to keep the most critical corridors open.
The extent and gravity of the COVID-19 pandemic caught the majority of governments in the world by surprise. The East Asian countries which were heavily affected by the 2003 SARS outbreak have now been reaping the benefits lessons learned from experience. But how does one replicate their strategies, built by years of preparation in a matter of weeks?
Collaboration. The extraordinary circumstance surrounding the current pandemic provides an ideal environment for collaboration and innovation. All the players in the knowledge value chain have a role to play: from simple data enumerators to highly-skilled data engineers, scientists and practitioners, citizens and policymakers. Big Data and AI capabilities are essential, but to leverage them for fighting the raging pandemic, what is needed is a collaborative mindset. •
References
Steel, John, and Anice C. Lowen. 2014. “Influenza: A Virus Reassortment.” In Influenza Pathogenesis and Control (Volume I), edited by Richard W. Compans and Michael B. A. Oldstone, 377–401. Cham: Springer International Publishing.